In an increasingly digital world, businesses rely on seamless online operations to meet customer demands, manage operations, and secure data.
But as the reliance on online platforms grows, so does the risk of cyberattacks, particularly Distributed Denial of Service (DDoS) attacks. These attacks, which flood servers with excessive requests, can lead to hours or even days of downtime, causing financial losses and damaging reputations. However, Artificial Intelligence (AI) has emerged as a powerful tool in the fight against DDoS attacks, enhancing prevention, detection, and response capabilities. Here’s how AI is reshaping the landscape of DDoS security and why businesses should take note.
Understanding the Threat: What Is a DDoS Attack?
A Distributed Denial of Service (DDoS) attack occurs when multiple devices, often infected with malware and grouped into a botnet, send a large volume of requests to a targeted server, application, or network.
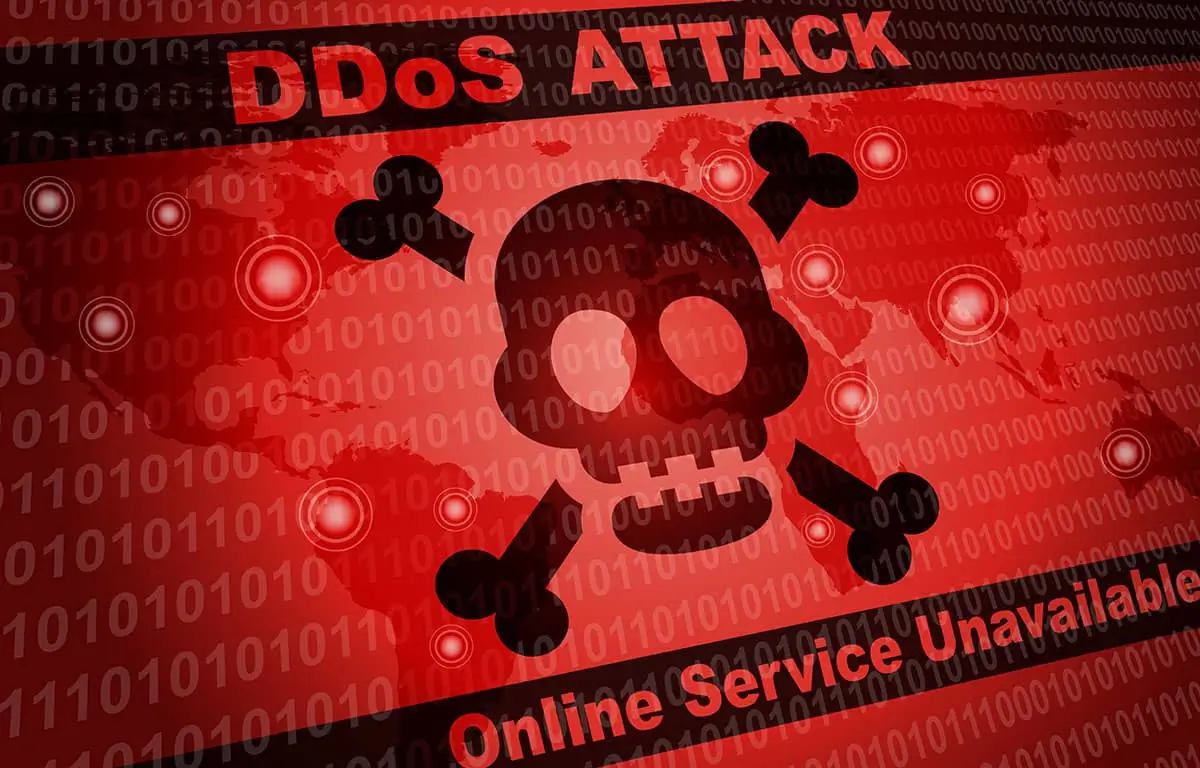
The goal is to overwhelm the system’s resources, causing slowdowns or crashes and rendering the service inaccessible to legitimate users.
These attacks can be difficult to detect manually, as they mimic legitimate user traffic and come from many different sources.
Traditional security measures often struggle to distinguish between real and malicious traffic, especially during complex, multi-vector DDoS attacks.
Why Traditional Methods Fall Short?
Traditional methods for DDoS prevention and detection involve in and out firewalls rule, rate-limiting, and manual monitoring. While these approaches offer some protection, they have significant limitations in handling the advanced, large-scale, and increasingly frequent DDoS attacks of today.
Manual methods are often too slow and lack the sophistication to detect evolving threats. Additionally, these measures cannot always differentiate between a real spike in legitimate traffic and a targeted DDoS attack.
This is where Artificial Intelligence comes in, providing a level of speed, accuracy, and adaptability that human operators and conventional tools cannot match.
How AI Enhances DDoS Prevention and Detection?
Artificial Intelligence offers several unique capabilities that make it well-suited for preventing and detecting DDoS attacks:
- Behavioral Analysis and Pattern Recognition: AI models can be trained to recognize normal user behavior patterns and distinguish them from potential DDoS attack patterns. By continuously monitoring network traffic and analyzing behavior in real time, AI can spot anomalies—such as sudden surges in traffic from specific regions or IP addresses—far quicker than traditional systems.
- Anomaly Detection: AI-powered systems use machine learning (ML) algorithms to establish a baseline of typical network activity. When traffic deviates significantly from this baseline, AI systems can immediately flag the deviation as suspicious. This approach not only helps detect ongoing attacks but can also prevent attacks by shutting down or limiting potentially malicious traffic.
- Real-Time Data Processing: AI-based systems are capable of processing and analyzing large volumes of data in real time, making them ideal for identifying attacks as they occur. This is especially important in the case of DDoS attacks, where every second counts. Real-time detection allows businesses to respond quickly, minimizing downtime and potential damage.
- Adaptive Learning: Unlike static security protocols, AI systems are continually learning and adapting. This adaptability means that AI can stay ahead of evolving attack tactics, adjusting its detection models based on new data and observed attack patterns. AI’s adaptive learning abilities also help in updating security measures to prevent similar attacks in the future.
Key AI Technologies in DDoS Mitigation.
Several AI technologies are playing a pivotal role in DDoS prevention and detection:
- Machine Learning (ML): ML algorithms, such as supervised, unsupervised, and deep learning models, analyze traffic patterns and detect deviations from normal behavior. Supervised ML models can be trained with labeled attack data to recognize specific attack signatures, while unsupervised models can identify unusual traffic without prior labeling, making them versatile for detecting novel attack methods.
- Neural Networks: Deep neural networks can analyze complex, multi-layered data to detect subtle patterns that might signal an attack. Their ability to manage vast amounts of data and detect intricate patterns makes them highly effective in DDoS detection.
- Natural Language Processing (NLP): In DDoS detection, NLP can be used to monitor communication patterns, such as those in real-time communication systems, chatbots, or social media, to anticipate and prevent attacks organized through these channels.
- Predictive Analytics: AI-based predictive models analyze historical data to identify trends that might precede a DDoS attack. This allows security teams to prepare for likely threats, allocate resources, and even initiate preventive measures before an attack occurs.
Advantages of AI in DDoS Prevention and Detection.
- Scalability: AI systems can handle large amounts of data, making them highly scalable solutions for DDoS detection across large networks and cloud environments. This is especially valuable for enterprises with complex, high-traffic infrastructure.
- Speed and Efficiency: AI operates at a speed and efficiency that humans cannot match, identifying and responding to potential threats in milliseconds. This rapid response time can be critical in mitigating the impact of an attack.
- Reduced False Positives: One of the challenges of DDoS detection is accurately identifying real threats without interrupting legitimate user traffic. AI’s pattern recognition and anomaly detection abilities help reduce false positives, ensuring that genuine traffic is not mistakenly blocked or slowed.
- Cost Savings: By automating the detection and response process, AI reduces the need for human intervention, ultimately saving time and costs associated with manual monitoring, analysis, and response.
Examples of AI in Action: Real-World Applications.
- Cloud-Based DDoS Protection: Many cloud providers, such as AWS, Google Cloud, and Microsoft Azure, have integrated AI-driven DDoS protection into their services. These systems monitor massive amounts of traffic data across their networks, applying AI algorithms to spot potential threats and initiate automatic responses, such as rerouting or throttling suspicious traffic.
- Security Information and Event Management (SIEM) Systems: SIEM systems like Splunk, IBM QRadar, and ArcSight now incorporate AI-driven analytics to identify suspicious patterns and provide alerts. These systems are widely used by security teams to detect and respond to DDoS attacks and other types of cyber threats.
- Botnet Detection: AI is also being used to detect botnet activity before it becomes a full-scale DDoS attack. By analyzing device behavior patterns, AI can identify devices that are part of a botnet and prevent them from launching an attack.
Challenges and Considerations in AI-Powered DDoS Defense.
While AI offers significant advantages, there are also challenges to consider:
- Resource Intensive: AI systems require substantial computational power, data storage, and high-quality training data, which can be costly to implement and maintain.
- Evolving Attack Techniques: Cyber attackers are constantly developing new tactics to evade detection. AI models need to be continuously updated and retrained to stay ahead of these evolving threats.
- Privacy Concerns: AI systems that monitor traffic in real time may inadvertently collect sensitive user data, raising privacy concerns. Ensuring compliance with data privacy regulations is essential.
The Future of AI in DDoS Defense.
Artificial intelligence’s role in DDoS prevention and detection is expected to grow as cyber threats become more sophisticated.
Future developments could see AI systems that operate autonomously, responding to threats without human intervention, or advancements in predictive analytics that allow organizations to preemptively block attacks before they begin.
Additionally, as AI-driven solutions become more accessible and cost-effective, we may see wider adoption, especially among small and medium-sized businesses that previously lacked the resources to implement robust DDoS defenses.
Conclusion.
Artificial Intelligence is transforming how we approach DDoS attack prevention and detection. With its ability to analyze vast amounts of data, detect anomalies in real time, and adapt to new threats, AI is a game-changer for organizations looking to protect themselves against the rising tide of DDoS attacks.
By investing in AI-driven solutions, businesses can ensure their operations remain secure, reliable, and resilient in the face of an ever-evolving cyber landscape.
Leave a Reply